Welcome to the
Chair of Systems Design
We are a group of about 15 researchers from various disciplines (statistical physics, applied mathematics, computer science, political science and economics). Our research can be best described as data driven modelling of complex systems with particular emphasis on social, socio-technical, and socio-economic systems.

Tracing Opioids Across the US: A High-Resolution Pharmaceutical Distribution Dataset
added

Empirical Networks are Sparse: Enhancing Multi-Edge Models with Zero-Inflation
added

Processing Large-Scale Archival Records: The Case of the Swiss Parliamentary Records
added
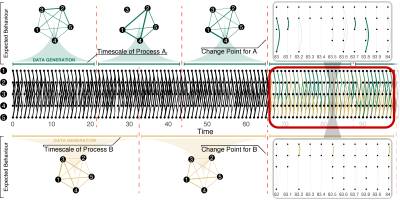
Disentangling the Timescales of a Complex System: A Bayesian Approach to Temporal Network Analysis
added
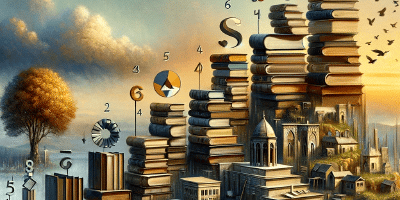
Talk: Rank Dynamics
Exploring rankings across systems, understanding adaptability and robustness.
added
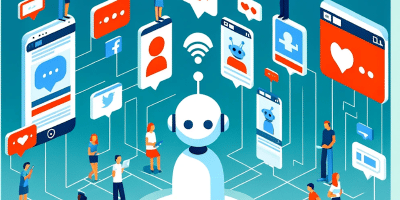
Talk: Large Language Models in the Age of Misinformation
added
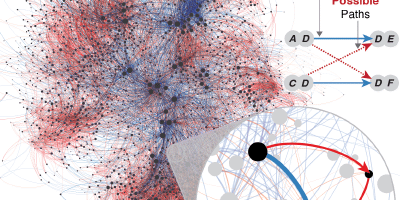
Paper on Supply Chain Resilience Published in Science Advances
Our research on pharmaceutical supply chain resilience has been published in …
added
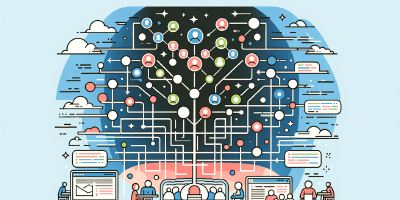
Event: Social Software Engineering
Symposium at ETH Zurich on December 15, 2023
added
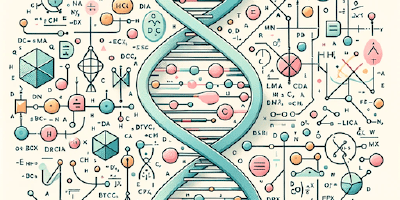
Talk by Rebekka Burkholz
Scaling Up Gene Regulatory Models to Fight Underspecification
added